Cybersecurity & AI
The Rise of AI-Powered Cyber Attacks: What Organizations Need to Know in 2024
Quest Lab Team • November 3, 2024 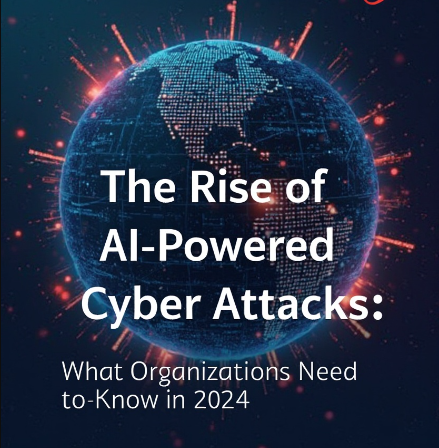
In recent years, Artificial Intelligence (AI) has made groundbreaking advancements across industries. However, while AI has revolutionized sectors like healthcare, finance, and manufacturing, it has also transformed cybersecurity landscapes—often with malicious consequences. AI-powered cyberattacks have emerged as a formidable threat, exploiting AI’s capabilities for creating, delivering, and concealing attacks with precision and speed. For organizations, understanding and preparing for these evolving threats is essential to safeguard data, protect privacy, and ensure business continuity.
AI-driven cyberattacks exploit machine learning, deep learning, and neural networks, enabling attackers to bypass traditional defenses, predict cybersecurity strategies, and learn from previous security measures. This article provides a deep dive into the mechanisms behind AI-powered cyberattacks, notable case studies, and practical defense strategies for organizations facing these new-age threats.
Understanding AI-Powered Cyber Attacks
AI-powered cyberattacks leverage the same AI technologies that benefit organizations—machine learning, data analytics, and pattern recognition—to enhance the precision, speed, and effectiveness of cyber attacks. These attacks can adapt to target systems' responses, learning in real time and adjusting strategies to optimize their impact.
How AI Transforms Traditional Attack Vectors
Attackers have adopted AI to escalate traditional methods such as phishing, malware, and Distributed Denial of Service (DDoS) attacks. By analyzing vast datasets and automating complex processes, AI allows these attack methods to become more difficult to detect and easier to execute on a massive scale. Machine learning algorithms, for instance, enable sophisticated social engineering attacks, crafting highly personalized phishing emails that are indistinguishable from legitimate communications.
Types of AI-Driven Cyber Attacks
Understanding the various types of AI-driven cyber attacks can help organizations recognize vulnerabilities within their systems. Below are some of the most prevalent AI-enabled attack types seen today:
1. AI-Enhanced Phishing Attacks
AI enhances traditional phishing by generating hyper-realistic and targeted phishing emails. AI algorithms analyze social media profiles, email correspondences, and digital footprints to craft personalized messages, increasing the likelihood of user interaction.
"Phishing campaigns are now capable of bypassing two-factor authentication by employing machine learning to predict user behavior and adapt responses in real-time."
2. Malware That Learns and Adapts
AI enables malware to evolve and adapt based on its environment, learning from security defenses and reconfiguring itself to avoid detection. For example, some AI-enabled malware hides its malicious payloads until specific conditions are met, minimizing the chance of being discovered during scanning.
3. AI-Driven Social Engineering
Social engineering attacks utilize AI to mimic human interactions convincingly, allowing attackers to deceive employees into divulging sensitive information. Advanced chatbots powered by natural language processing (NLP) can hold human-like conversations, posing as trusted contacts to manipulate users.
Case Studies of AI-Enabled Cyber Attacks
Examining real-world examples of AI-powered cyberattacks highlights how these threats manifest in actual scenarios. These cases underscore the importance of AI-aware security practices:
Case 1: AI-Powered Spear Phishing in Finance
A major financial institution faced a spear phishing attack where attackers used machine learning to mimic the email style of senior executives. By analyzing internal communications, AI algorithms generated messages that perfectly mirrored company-specific language and formatting, successfully deceiving employees into transferring funds to unauthorized accounts.
Case 2: Malware Evasion Through AI in Healthcare
Healthcare organizations, often vulnerable due to outdated infrastructure, have become prime targets for AI-driven malware. One ransomware attack utilized machine learning to hide malicious code within legitimate medical software. The malware adapted in response to anti-virus scans, avoiding detection and ultimately locking down patient data.
Defensive Strategies Against AI-Driven Attacks
Organizations need a multi-layered defense approach to counteract the adaptability and precision of AI-enabled threats. Below are recommended strategies for mitigating these attacks:
Adopting AI for Threat Detection
Using AI for cybersecurity can empower threat detection systems with anomaly detection, predictive analysis, and real-time response capabilities. AI-driven tools can rapidly identify unusual patterns in network traffic, alerting security teams to potential attacks before they escalate.
Best Practices for AI-Based Defense Systems
Implementing AI-based defenses requires careful planning and expert oversight:
- Invest in continuous training of machine learning models to recognize evolving attack patterns.
- Ensure human oversight of AI systems to review flagged anomalies.
- Integrate behavioral analysis tools that detect deviations from normal network behavior.
Strengthening Employee Training and Awareness
Educating employees on AI-enhanced phishing and social engineering tactics is essential. Organizations should invest in regular training programs, educating employees on how to identify AI-manipulated content and recognize warning signs of sophisticated scams.
As the landscape of technology rapidly advances, so too does the sophistication of cyber threats. Traditional attacks like phishing and malware are being augmented by Artificial Intelligence (AI), allowing attackers to increase the precision, speed, and efficacy of their attacks. AI-driven cyber threats are uniquely challenging due to their adaptability and ability to learn from defensive measures, making them far more resilient against traditional security tactics.
According to cybersecurity firm Darktrace, AI-based threats have increased by 400% over the past two years. These attacks vary in nature but share a common feature: they are powered by data-driven machine learning models that allow attackers to bypass conventional security protocols. Organizations across various industries, from finance to healthcare, are feeling the effects as attackers use AI to create tailored, resilient, and highly deceptive attacks.
Evolving Threat Landscape with AI-Powered Attacks
Traditional cybersecurity relies heavily on signature-based detection, where specific patterns or behaviors associated with malicious activities trigger an alarm. However, AI-powered attacks bypass these defenses by adapting their signatures dynamically. With machine learning, attackers can evade these traditional detection systems, leaving organizations vulnerable to breaches they may not even be able to detect until it’s too late.
AI-Driven Ransomware: A Persistent Threat
Ransomware is one of the most severe threats exacerbated by AI. In 2023, a major hospital in Germany experienced an AI-driven ransomware attack that led to a complete shutdown of its operations, costing millions in losses and leading to delays in patient care. Attackers used machine learning to identify critical infrastructure, avoiding non-essential systems and thus maximizing their impact. This level of specificity and strategic targeting is only possible through AI-enabled reconnaissance.
"The future of ransomware is adaptive and personalized, enabled by AI’s ability to analyze data and predict system vulnerabilities with unprecedented accuracy."
Deepfake-Assisted Social Engineering
Deepfake technology, powered by deep learning algorithms, has become a powerful tool for social engineering. In 2022, an AI-based deepfake scam targeted a UK-based energy firm. Attackers mimicked the CEO’s voice using AI voice synthesis, convincing an employee to transfer $240,000 to a fraudulent account. The impersonation was so convincing that traditional security checks failed to flag the transaction as suspicious.
With deepfake technology, attackers can easily replicate voices, faces, and other personal identifiers. This poses a significant challenge as these attacks exploit trust within organizations, making it crucial for companies to adopt advanced verification measures beyond just voice or visual recognition.
Advanced Techniques Used in AI-Powered Attacks
AI-driven attacks often employ a combination of machine learning, natural language processing (NLP), and behavioral analysis. These technologies enable attackers to analyze vast datasets to predict behavior patterns, discover vulnerabilities, and automate attacks at a scale previously unimaginable.
Predictive Behavioral Analysis
Predictive behavioral analysis involves training models on historical data to predict and manipulate future behaviors. For instance, attackers use behavioral patterns in phishing emails to adapt messages based on user reactions, resulting in higher engagement and success rates. AI can adjust email language, timing, and even content based on individual user behavior, making it increasingly difficult for users to distinguish between legitimate and malicious communications.
Code Obfuscation and Polymorphic Malware
Polymorphic malware, which changes its code structure to evade detection, has become more powerful with AI. Attackers use deep learning models to automatically obfuscate malicious code, making it undetectable by traditional antivirus software. This adaptive approach to code structure allows malware to persist on systems without triggering standard security alerts.
Proactive Defense Strategies for Organizations
Organizations facing AI-powered cyber threats must adopt a proactive approach, incorporating both technological and human-centric strategies to counteract these sophisticated attacks. While traditional defense measures remain essential, new approaches must integrate machine learning, real-time monitoring, and employee training.
Leveraging AI for Threat Detection and Incident Response
Using AI in defense systems can turn the tables on attackers. For example, anomaly detection algorithms powered by machine learning can identify unusual behavior on networks, such as large, unexpected data transfers, and trigger automated alerts for immediate investigation. These systems continuously learn from the behavior of legitimate users, improving detection capabilities over time.
Core Elements of an AI-Driven Defense System
Organizations should ensure their AI defense strategy includes the following elements:
- Automated response protocols to contain threats at the earliest detection point.
- Continuous model updates to adapt to emerging threat patterns.
- Collaborative analysis with threat intelligence platforms for shared insights.
Human-Centric Approaches: Training and Awareness
The human element remains critical in defense. Training employees to identify AI-driven phishing attempts, deepfakes, and social engineering tactics can significantly reduce the risk of successful attacks. Companies like Google and Microsoft have incorporated simulation-based training to help employees recognize malicious content and understand the tactics used in AI-driven social engineering.
Incorporating Multi-Factor Authentication and Biometric Checks
Relying solely on passwords for authentication leaves systems vulnerable to AI-driven attacks that can easily break traditional credentials. Multi-factor authentication (MFA) and biometric checks offer enhanced security layers. Advanced biometric systems use AI to monitor user behavior over time, identifying anomalies that may indicate unauthorized access.
The Road Ahead: Future Trends in AI and Cybersecurity
Looking to the future, the interplay between AI and cybersecurity will continue to grow more complex. Quantum computing, which promises unprecedented computational power, could make AI-driven attacks even faster and more sophisticated. Similarly, developments in autonomous machine learning models—systems that evolve without human input—could create new attack methods that are currently unimaginable.
According to a study by Gartner, organizations that do not incorporate AI-based defense systems may face a 60% higher risk of data breaches by 2025. This is a clear call for businesses to begin implementing AI not only as a protective measure but as a proactive approach to cybersecurity, anticipating and addressing threats before they arise.
- Embrace adaptive AI: Leverage AI solutions that evolve with emerging threats, incorporating them into broader security frameworks.
- Focus on resilience: A robust incident response plan can limit damage during an AI-driven attack and expedite recovery.
- Collaborate for intelligence sharing: Partnering with other organizations to share threat intelligence data can help preemptively block AI-driven attacks.
Ultimately, AI-powered cyberattacks represent a transformative shift in the cybersecurity landscape. The speed and scale of these threats call for an equally sophisticated response, driven by AI and supported by informed, proactive strategies. As AI continues to evolve, organizations must remain vigilant, adapting their defenses and fostering a culture of awareness to stay ahead in the ever-changing cybersecurity landscape.
AI-Driven Attacks on Critical Infrastructure
In recent years, there has been a marked increase in AI-driven attacks targeting critical infrastructure. One notable example is the 2023 attack on a power grid in Eastern Europe. Attackers used AI algorithms to study power distribution patterns, identifying optimal times to launch an attack that would maximize disruption. The AI system learned from previous attempts, bypassing detection tools and exploiting vulnerabilities in operational technology systems.
"The AI-powered attack on our grid demonstrated an unprecedented level of adaptability. The system adjusted in real-time to avoid our defenses." - Chief Information Security Officer, Anonymous Energy Provider
In response, several governments have ramped up cybersecurity measures for critical infrastructure. In the United States, the Cybersecurity and Infrastructure Security Agency (CISA) introduced AI-specific protocols to detect anomalies in utility operations. These protocols require machine learning models that continuously monitor infrastructure behavior to identify deviations potentially linked to malicious activities.
How AI Enhances Phishing Attacks
Phishing attacks have been a long-standing cyber threat, but AI has taken these attacks to a new level. AI algorithms can tailor phishing emails with remarkable accuracy, analyzing data from social media, emails, and professional networks to create messages that appear highly credible to recipients. This level of personalization is not achievable through manual tactics alone.
A high-profile example occurred in 2023, when AI-powered phishing emails successfully targeted employees at a major financial institution. The AI crafted emails based on recent interactions, leveraging data to mimic internal communications accurately. Employees reported that the emails closely resembled official communications, containing details like recent projects and organizational language style, which increased the success rate of the phishing attempt.
The Role of NLP in AI-Powered Phishing
Natural Language Processing (NLP) models play a crucial role in crafting convincing phishing emails. AI systems analyze the text to generate adaptive language, tailoring the tone, style, and content based on prior interactions and recipient behavior. Security experts now recognize the need for advanced AI-based defense mechanisms to detect and block NLP-enhanced phishing attempts in real-time.
Regulatory Responses to AI-Powered Threats
As AI-based threats become more prevalent, regulatory bodies worldwide are implementing measures to mitigate these risks. The European Union’s Digital Operational Resilience Act (DORA), for instance, includes provisions that mandate organizations to report AI-driven security incidents, particularly those affecting financial institutions. By requiring immediate reporting, DORA aims to enhance the rapid exchange of threat intelligence across sectors.
Similarly, in the United States, the National Institute of Standards and Technology (NIST) introduced guidelines to assist companies in identifying, protecting, detecting, and responding to AI-driven attacks. These guidelines emphasize continuous monitoring, AI model validation, and regular assessment of AI-driven vulnerabilities, creating a foundation for defensive AI technology.
Ethics and AI in Cybersecurity
The development and deployment of AI in cybersecurity raise critical ethical questions. Cybersecurity professionals face dilemmas regarding the balance between AI-powered defenses and potential misuse of AI for offensive purposes. Ethics frameworks, such as the IEEE's Ethically Aligned Design, encourage developers to create AI with 'do no harm' principles, prioritizing transparency, fairness, and accountability.
"Ethical considerations are paramount in AI cybersecurity. AI should protect, not harm, yet its dual-use nature makes regulation essential to prevent misuse." - Professor John Keller, Cybersecurity Ethics Specialist
Strategic Collaborations to Combat AI Threats
Given the complexity and adaptability of AI-driven cyber threats, collaboration among organizations, cybersecurity firms, and governments is essential. The Global Forum on Cyber Expertise (GFCE) has launched initiatives encouraging countries to share data on AI-based attacks, establishing protocols that allow for swift countermeasures.
Companies such as Microsoft, IBM, and Google are collaborating on open-source projects that aim to build defensive AI tools accessible to smaller organizations. These tools leverage machine learning to identify anomalies in network traffic, authenticate user behavior, and detect data exfiltration attempts, providing a critical defense layer for organizations lacking extensive cybersecurity resources.
Challenges and Limitations of Defensive AI
While defensive AI holds promise, it faces limitations and challenges. Machine learning models are only as effective as the data they are trained on, and adversarial attacks can exploit weaknesses in these models. For example, attackers have successfully launched evasion attacks on defensive AI by introducing perturbations, slight modifications to data inputs that fool detection algorithms without triggering alarms.
One high-profile instance of adversarial attacks involved researchers manipulating an image recognition AI to misidentify objects by adding inconspicuous 'noise' to images. This type of attack has implications in cybersecurity, where attackers can disguise malicious actions to evade AI-based defenses. To counter these tactics, cybersecurity experts are exploring techniques like adversarial training, which involves training models on altered data to recognize subtle patterns in adversarial behavior.
Future-Proofing Cybersecurity Against AI-Driven Threats
As AI continues to evolve, so will the threat landscape. Cybersecurity firms and organizations must focus on building resilience, investing in continuous improvement of defense capabilities, and adopting a flexible, adaptive approach to security. Future-proofing cybersecurity involves adopting robust security frameworks, encouraging collaboration between public and private sectors, and promoting a culture of continuous learning within organizations.
- Embrace resilience frameworks: Leveraging models like NIST’s Cybersecurity Framework can provide a strategic approach to manage and reduce risks.
- Engage in proactive threat hunting: AI-driven threat hunting initiatives can help detect AI-based attacks before they cause damage.
- Prioritize workforce education: Training teams on AI-based threat patterns, including how to recognize sophisticated phishing and deepfakes, remains vital.
AI-powered cyber attacks represent a transformative shift in the cybersecurity domain. The rapid advancement of AI technology will bring both innovative defensive measures and novel attack vectors. Organizations must remain vigilant and adaptive, equipping themselves with advanced tools, knowledge, and collaborative networks to effectively navigate the challenges posed by AI-driven threats.
Future of AI in Cybersecurity: Opportunities and Risks
As AI technology advances, both its potential for cyber defense and exploitation will grow. Quantum computing, another emerging technology, could potentially make AI algorithms faster and more powerful, raising new concerns about cybersecurity. Moving forward, organizations will need to continually adapt and integrate AI defensively to stay one step ahead of attackers.
Organizations must strike a balance between leveraging AI for cybersecurity benefits while addressing its potential risks. Ultimately, a proactive approach—integrating AI defensively, educating stakeholders, and staying vigilant against AI-driven threats—will help organizations safeguard their digital assets and build resilience against future cyber threats.
Quest Lab Writer Team
This article was made live by Quest Lab Team of writers and expertise in field of searching and exploring
rich technological content on Cybersecurity and its future with its impact on the modern world