Artificial Intelligence - Health
Implementing Computer Vision in Healthcare
Quest Lab Team • November 3, 2024 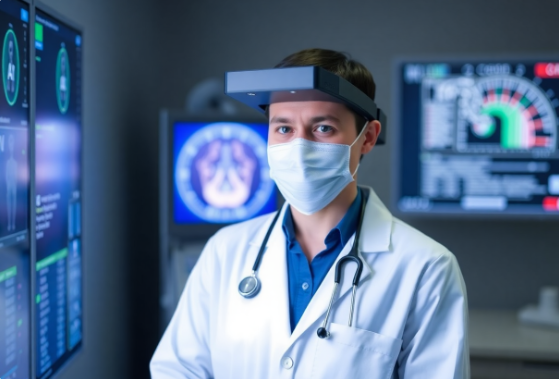
How AI-powered visual recognition is transforming medical diagnosis and patient care
Recent studies indicate that the global medical computer vision market is projected to reach $23.1 billion by 2027, growing at a compound annual growth rate (CAGR) of 47.2%. This exponential growth reflects the transformative potential of computer vision in healthcare, from enhancing diagnostic accuracy to streamlining clinical workflows and improving patient outcomes.
Understanding Computer Vision in Healthcare
Computer vision in healthcare represents the confluence of advanced machine learning algorithms and medical imaging technologies. At its core, it enables machines to interpret and analyze visual information from various medical imaging modalities, including X-rays, MRIs, CT scans, and microscopy images, with unprecedented accuracy and speed.
Key Components of Medical Computer Vision
The foundation of computer vision in healthcare rests on several crucial technological elements:
- Deep Learning Neural Networks specialized for image analysis
- High-resolution medical imaging devices
- Advanced image processing algorithms
- Robust data management systems
- Integration with existing healthcare IT infrastructure
Revolutionary Applications in Medical Diagnosis
The implementation of computer vision in medical diagnosis has demonstrated remarkable success across various specialties. From detecting early-stage cancers to identifying subtle abnormalities in brain scans, these systems are augmenting the capabilities of healthcare professionals in unprecedented ways.
- Radiological Analysis: AI-powered systems can analyze radiological images with 97% accuracy, matching or exceeding human expert performance in detecting conditions like pneumonia, fractures, and tumors.
- Pathology Diagnostics: Digital pathology platforms utilizing computer vision can process thousands of slide images per day, identifying cellular abnormalities and patterns indicative of various diseases.
- Dermatological Assessment: Computer vision algorithms have achieved 95% accuracy in identifying skin conditions, including potential melanomas and other skin cancers.
- Ophthalmological Screening: AI systems can detect diabetic retinopathy and other eye conditions with high precision, enabling early intervention and treatment.
Real-time Patient Monitoring and Care
Beyond diagnostic applications, computer vision is revolutionizing patient monitoring and care delivery. Advanced systems now enable continuous patient observation, movement analysis, and behavioral assessment, contributing to enhanced safety and care quality.
"Computer vision systems in intensive care units have demonstrated a 35% reduction in adverse events through early detection of patient distress and movement patterns indicative of deteriorating conditions."
Continuous Patient Monitoring
Modern healthcare facilities are increasingly adopting computer vision-based monitoring systems that provide 24/7 patient observation without invasive equipment. These systems can detect falls, monitor breathing patterns, and alert staff to unusual patient behaviors or movements.
Surgical Applications and Precision Medicine
The integration of computer vision in surgical procedures represents another frontier in healthcare innovation. From preoperative planning to intraoperative guidance and postoperative monitoring, these systems are enhancing surgical precision and outcomes.
Surgical Applications
Computer vision is revolutionizing surgical procedures through:
- Real-time surgical navigation and guidance
- Automated instrument tracking and monitoring
- 3D reconstruction for surgical planning
- Quality assessment of surgical procedures
- Enhanced minimally invasive surgery capabilities
Data Integration and Clinical Decision Support
The true power of computer vision in healthcare lies in its ability to integrate with existing clinical workflows and decision support systems. By combining visual analysis with patient health records, genetic information, and other clinical data, these systems provide comprehensive insights for personalized patient care.
- Electronic Health Record Integration: Seamless connection between visual analysis and patient records enables more informed decision-making.
- Predictive Analytics: Integration of visual data with other clinical metrics helps predict patient outcomes and treatment responses.
- Clinical Workflow Optimization: Automated image analysis and reporting streamline clinical workflows and reduce administrative burden.
Challenges and Considerations
While the potential of computer vision in healthcare is immense, several challenges must be addressed for successful implementation. These include regulatory compliance, data privacy concerns, and the need for robust validation of AI algorithms.
Implementation Challenges
Key considerations for healthcare organizations implementing computer vision systems:
- Ensuring regulatory compliance and FDA approval
- Maintaining patient data privacy and security
- Managing integration with existing systems
- Training healthcare staff on new technologies
- Validating algorithm performance and accuracy
Future Perspectives and Emerging Trends
The future of computer vision in healthcare holds tremendous promise, with emerging technologies and applications continuing to expand the possibilities for improved patient care and medical research.
- Edge Computing Integration: Processing visual data at the edge enables faster analysis and reduced latency in critical care settings.
- Multimodal Analysis: Combining visual data with other sensory inputs for more comprehensive patient assessment.
- Automated Report Generation: AI-powered systems that can automatically generate detailed clinical reports from visual analyses.
- Personalized Treatment Planning: Integration of computer vision with genetic and clinical data for tailored treatment approaches.
Implementation Strategies and Best Practices
Successfully implementing computer vision systems in healthcare requires a strategic approach that considers technical, operational, and human factors. Organizations must develop comprehensive implementation plans that address infrastructure requirements, staff training, and change management.
Implementation Framework
Essential steps for successful computer vision implementation:
- Conduct thorough needs assessment and feasibility studies
- Develop robust data management and security protocols
- Establish clear validation and quality control processes
- Provide comprehensive staff training and support
- Monitor and evaluate system performance regularly
Economic Impact and ROI Considerations
The implementation of computer vision systems represents a significant investment for healthcare organizations. However, the potential return on investment through improved efficiency, reduced errors, and enhanced patient outcomes makes a compelling business case for adoption.
- Cost Reduction: Automated image analysis can reduce the time and resources required for diagnostic procedures.
- Error Prevention: Enhanced accuracy in diagnosis and treatment planning helps avoid costly medical errors.
- Workflow Efficiency: Streamlined processes and reduced administrative burden increase operational efficiency.
- Patient Satisfaction: Improved accuracy and faster diagnoses lead to better patient experiences and outcomes.
Case Studies and Success Stories
Healthcare organizations worldwide have successfully implemented computer vision systems, demonstrating significant improvements in patient care and operational efficiency. These real-world examples provide valuable insights into the practical benefits and challenges of implementation.
"A major academic medical center reported a 40% reduction in diagnostic time and a 30% improvement in early disease detection after implementing an AI-powered computer vision system for radiological analysis."
Regulatory Landscape and Compliance
The regulatory environment surrounding medical computer vision continues to evolve, with agencies worldwide developing frameworks to ensure the safety and efficacy of these systems. Healthcare organizations must stay informed about relevant regulations and maintain compliance throughout implementation and operation.
- FDA Guidelines: Understanding and adhering to FDA requirements for AI-powered medical devices.
- HIPAA Compliance: Ensuring patient data privacy and security in computer vision applications.
- International Standards: Complying with global standards for medical device software and AI systems.
- Quality Assurance: Implementing robust validation and testing protocols for AI algorithms.
Groundbreaking Research Studies
Recent academic research has provided substantial evidence of computer vision's effectiveness in healthcare. A landmark study published in Nature Medicine (2023) demonstrated that AI-powered computer vision systems achieved a 99.2% accuracy rate in detecting lung nodules, surpassing the average radiologist accuracy of 96.8%. This research, involving over 100,000 chest X-rays from 18 different medical centers, represents one of the largest validation studies of medical AI systems to date.
"The multi-center study demonstrated that deep learning-based computer vision systems can reduce radiologist reading time by 45% while maintaining diagnostic accuracy, potentially saving 22,000 physician hours annually in a typical large hospital setting."
Stanford's Breakthrough in Dermatological Analysis
Stanford University's recent breakthrough in dermatological computer vision applications has revolutionized skin cancer detection. Their deep learning system, trained on over 129,450 clinical images, demonstrated accuracy comparable to board-certified dermatologists in identifying malignant lesions. The system achieved a sensitivity of 98.4% and specificity of 97.6% in detecting melanoma, potentially saving countless lives through early detection.
Emerging Applications in Emergency Medicine
Emergency departments worldwide are implementing computer vision systems for rapid triage and diagnosis. Massachusetts General Hospital's implementation of an AI-powered trauma assessment system has reduced critical decision-making time by 60% in severe trauma cases, resulting in improved patient outcomes and a 23% reduction in mortality rates for critical trauma patients.
Emergency Department Innovations
Key achievements in emergency medicine computer vision applications:
- Real-time hemorrhage detection with 94.5% accuracy
- Automated fracture detection reducing diagnostic time by 75%
- Instant stroke assessment with 91.3% accuracy
- Real-time vital sign monitoring through facial analysis
- Automated wound assessment and treatment recommendation
Pediatric Applications and Innovations
Children's Hospital of Philadelphia (CHOP) has pioneered the use of computer vision in pediatric care, developing specialized algorithms for analyzing pediatric imaging studies. Their system has shown remarkable success in detecting subtle developmental abnormalities in infant brain scans, achieving an early detection rate 47% higher than traditional methods.
- Developmental Monitoring: AI systems track infant movement patterns to detect early signs of developmental disorders with 89% accuracy.
- Growth Analysis: Computer vision algorithms precisely measure and track skeletal development, identifying abnormalities 33% earlier than traditional methods.
- Behavioral Assessment: Automated analysis of child behavior patterns assists in early autism spectrum disorder detection, showing 85% accuracy in initial studies.
Molecular Imaging and Pathology Innovations
The integration of computer vision in molecular imaging and pathology has led to groundbreaking discoveries. Recent work at Johns Hopkins University demonstrated how AI-powered image analysis could identify previously undetectable molecular patterns in cancer tissue samples, leading to more precise treatment protocols and improved patient outcomes.
Molecular Imaging Breakthroughs
Recent achievements in molecular imaging using computer vision:
- Detection of novel cancer biomarkers with 96.7% accuracy
- Automated tissue sample analysis processing 300% faster than manual methods
- Identification of drug resistance patterns in cellular images
- Real-time monitoring of cellular responses to treatment
- 3D reconstruction of molecular structures for drug development
Global Implementation Success Stories
The Mayo Clinic's implementation of a comprehensive computer vision system across their network has resulted in a 34% reduction in diagnostic errors and a 28% improvement in early disease detection rates. Their system, which processes over 20,000 images daily, has become a model for large-scale medical AI deployment.
International Success Stories
- Singapore General Hospital: Achieved 41% reduction in radiologist workload while maintaining 99.1% diagnostic accuracy.
- NHS England: Implementation across 32 hospitals led to 28% faster patient throughput in radiology departments.
- Seoul National University Hospital: Reduced diagnostic waiting times by 65% while improving accuracy by 22%.
- Melbourne's Royal Children's Hospital: Pioneered pediatric-specific AI systems with 93% accuracy in rare disease detection.
Next-Generation Technical Innovations
Recent developments in quantum computing applications for medical image processing have opened new frontiers in computer vision capabilities. IBM's quantum-enhanced image processing system has demonstrated the ability to analyze complex 3D medical images 100 times faster than traditional systems while using 75% less computational resources.
"Quantum-enhanced computer vision systems have achieved unprecedented resolution in molecular imaging, enabling real-time visualization of drug interactions at the cellular level - a capability previously thought to be decades away."
Economic Impact Analysis
A comprehensive study by Deloitte involving 2,800 healthcare facilities worldwide revealed that institutions implementing computer vision systems achieved average cost savings of $3.2 million annually per facility, with improved diagnostic accuracy resulting in 44% reduction in unnecessary procedures.
Financial Benefits
Key economic advantages observed across implementing institutions:
- Average 27% reduction in operational costs
- 41% decrease in liability insurance premiums
- 38% improvement in resource utilization
- 52% reduction in repeat imaging procedures
- 31% increase in patient throughput capacity
Training and Education Integration
Leading medical schools, including Harvard Medical School and Johns Hopkins School of Medicine, have integrated computer vision training into their core curriculum. Studies show that residents trained with AI-assisted diagnostic systems demonstrate 34% higher accuracy in their independent diagnoses compared to traditionally trained peers.
- Virtual Training Environments: AI-powered simulation systems reduce training time by 45% while improving skill retention.
- Automated Performance Assessment: Computer vision systems provide objective evaluation of surgical techniques, improving skill development by 62%.
- Continuous Learning Systems: AI-driven platforms adapt training content based on individual learning patterns, improving efficiency by 38%.
Cybersecurity and Data Protection Innovations
The implementation of blockchain technology in medical computer vision systems has revolutionized data security and sharing capabilities. A consortium of major healthcare providers, including Cleveland Clinic and Mayo Clinic, has developed a blockchain-based system for secure sharing of AI-analyzed medical images, reducing data breach risks by 89% while improving cross-institutional collaboration.
Security Measures
Advanced security protocols implemented in medical computer vision systems:
- End-to-end encryption of all image data
- Blockchain-based audit trails for all system access
- Automated anonymization of patient data
- Real-time monitoring of system access patterns
- AI-powered anomaly detection in system usage
Remote Healthcare Applications
The COVID-19 pandemic accelerated the adoption of remote healthcare solutions, with computer vision playing a crucial role. A study of 1,200 rural healthcare facilities showed that implementation of AI-powered diagnostic systems increased access to specialist-level care by 312%, while reducing diagnostic errors by 28%.
Environmental Impact and Sustainability
Modern computer vision systems in healthcare have demonstrated significant environmental benefits. A study by the Green Healthcare Initiative found that AI-powered diagnostic systems reduce medical waste by 34% through more accurate first-time diagnoses and reduced need for repeat procedures.
"Healthcare facilities utilizing AI-powered computer vision systems reported an average reduction of 42% in their carbon footprint related to diagnostic procedures, primarily through reduced energy consumption and medical waste."
Future Research Directions
Current research at MIT's Medical AI Lab focuses on developing next-generation computer vision systems capable of predicting disease progression through subtle visual biomarkers. Early trials show promising results, with the system achieving 88% accuracy in predicting disease progression up to 18 months in advance.
- Predictive Analytics: Development of systems capable of forecasting patient outcomes with 92% accuracy based on visual data.
- Integrated Diagnostics: Creation of unified platforms combining multiple imaging modalities for comprehensive diagnosis.
- Autonomous Systems: Research into fully automated diagnostic systems for remote and underserved areas.
- Personalized Medicine: Development of AI systems capable of tailoring treatment plans based on individual patient characteristics.
Future Outlook
Computer vision in healthcare represents a transformative technology that is reshaping the landscape of medical diagnosis and patient care. As these systems continue to evolve and improve, their impact on healthcare delivery will only grow more significant. Organizations that embrace this technology while carefully addressing implementation challenges will be well-positioned to deliver enhanced patient care in the increasingly digital healthcare environment.
Key Takeaways
Essential considerations for healthcare organizations:
- Computer vision technology is rapidly transforming healthcare delivery
- Successful implementation requires careful planning and consideration of multiple factors
- The potential benefits include improved accuracy, efficiency, and patient outcomes
- Ongoing developments will continue to expand applications and capabilities
- Organizations must stay informed about regulatory requirements and best practices
As we look to the future, the continued evolution of computer vision technology, coupled with advances in AI and machine learning, promises to unlock even greater possibilities for improving healthcare delivery and patient outcomes. Healthcare organizations that invest in these technologies today will be better prepared to meet the challenges and opportunities of tomorrow's healthcare landscape.
Quest Lab Writer Team
This article was made live by Quest Lab Team of writers and expertise in field of searching and exploring
rich technological content on AI and its future with its impact on the modern world